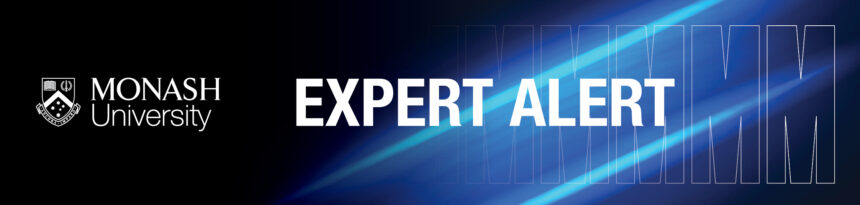
A Monash University expert is available to comment on the generation of deepfakes during election campaigns, how to detect deepfakes and what cautionary measures can be taken against AI-generated misinformation.
Associate Professor Abhinav Dhall, Department of Data Science & AI, Faculty of Information Technology
Contact details: +61 450 501 248 or media@monash.edu
Human-centred artificial intelligence
Audio-visual deepfakes
Computer vision
The following can be attributed to Associate Professor Dhall:
“The use of generative AI makes it easier for legitimate election campaigning content to be generated but it also makes it easier and faster for miscreants to generate and spread misinformation or disinformation, as we have seen during elections across the globe recently in the United States and India.
“AI-generated audio and video deepfakes are commonly distributed through social media and chat platforms such as X, Facebook, Instagram, TikTok, WhatsApp and others. They spread rapidly due to algorithm-driven recommendations and mass sharing.
“Most social media platforms do not check if an audio, image or video is a deepfake when the content is being uploaded to their platform. This is an important step to curb the spread of deepfakes. While some platforms are investing in detection tools, enforcement remains inconsistent. It is now important to cross-check information across multiple trusted media and platforms that use appropriate validation tools.
“Deepfake generation programs are available as apps and open source tools. With this a perpetrator can create high quality deepfakes in multiple languages. But thankfully, current deepfakes detectors are improving rapidly as well, and can detect fakes generated from a wide variety of generative AI methods.
“In some cases it is possible to detect deepfakes. This kind of content generation software often leaves subtle flaws in both audio and visual details. By closely examining a video, viewers may notice inconsistencies such as poor lip synchronisation, missing teeth, unnatural eye blinking, uneven lighting on the face, or a lack of facial expressions. Similarly, audio may contain artefacts such as a robotic sounding voice or a lack of natural emotion, which can indicate that the video may be a deepfake.
“Videos that blend real, unaltered footage with deepfake content are significantly harder for viewers to detect. Even minor alterations, such as changing specific words in a speech, can completely distort the meaning of a statement, making the manipulation more convincing. These types of deepfakes pose a greater challenge as they exploit genuine elements to enhance credibility, making detection and verification even more difficult. Current research is looking to develop solutions for these complex scenarios.”
For any other topics on which you may be seeking expert comment, contact the Monash University Media Unit on +61 3 9903 4840 or media@monash.edu